Today, data science has become a game-changer for various sectors, and retail is no exception. In an ever-evolving digital world, using the power of data science has become vital for retailers trying to remain ahead of the competition. By utilizing the massive quantity of data accessible, merchants can improve the consumer experience and drive sales like never before. In this blog article, we will look into the numerous ways in which data science is altering the retail industry and investigate how merchants may employ this technology to streamline their operations, customize marketing efforts, and ultimately increase their bottom line. From predictive analytics to recommender systems, let’s unearth the huge potential of Data Science in Retail.

Table of Contents
Introduction to data science in retail
Data science has changed several sectors, and retail is no exception. With the large quantity of data created in the retail industry, organizations have begun embracing data science approaches to gather important insights and make educated choices. In this post, we will investigate the importance of data science in retail and how it is revolutionizing the way merchants operate.
In the retail business, data science entails obtaining, analyzing, and interpreting large volumes of data to find patterns, trends, and consumer behavior. By leveraging complex algorithms and statistical models, merchants may extract relevant insights from data and make data-driven choices.
The role of data science in enhancing customer experience
Data science plays a key role in increasing the consumer experience across numerous businesses, not just in retail. By integrating data analytics, machine learning, and artificial intelligence, firms may obtain deep insights into consumer behavior, preferences, and pain areas, leading to enhanced goods, services, and interactions. Here are some significant ways data science impacts the consumer experience:
- Personalization: Data science helps firms to develop individualized experiences suited to each consumer. By evaluating client data, including demographics, purchase history, browsing activity, and social media interactions, organizations may send personalized suggestions, offers, and content that connect with individual tastes. This degree of personalization promotes consumer happiness and loyalty.
- Predictive Analytics: Data science allows firms to apply predictive models to anticipate consumer demands and behavior. By evaluating historical and real-time data, firms may estimate client preferences, detect future churn, and proactively provide appropriate solutions. This helps firms remain one step ahead and create individualized experiences, eventually boosting customer happiness and retention.
- Client Segmentation: Data science approaches enable firms to segment their client base into relevant groups based on multiple characteristics such as demographics, behavior, and preferences. This segmentation helps organizations adjust their marketing efforts and product offers to particular consumer categories, resulting in more focused and successful customer experiences.
- Chatbots and Virtual Assistants: Data science-powered chatbots and virtual assistants give clients rapid and tailored help. These chatbots may assess client inquiries, prior encounters, and data from many sources to give accurate and useful replies, resulting in speedier problem resolution and better customer satisfaction.
- Customer Sentiment Analysis: With data science, firms may examine customer feedback, social media mentions, and online reviews to acquire insights into consumer sentiment. Sentiment analysis tools assist firms in understanding how consumers view their goods, services, and brands, enabling them to make required modifications and address any issues swiftly.
- Continuous Improvement: Through data science, firms may acquire and evaluate consumer feedback, use trends, and performance indicators to discover areas for development. By finding trends and insights, firms can iterate and upgrade their goods, services, and customer experiences, ensuring they match shifting consumer expectations.
Data science helps firms obtain a greater knowledge of their consumers, allowing them to create tailored experiences, anticipate demands, and constantly enhance goods and services. By embracing the power of data, firms can better the customer experience, develop deeper connections, and drive corporate success.
How data science drives sales in retail
Data science plays a significant role in generating sales in the retail business. By embracing the power of data analytics and predictive modeling, retailers can make educated choices and improve their operations to enhance the consumer experience. Here are some ways data science promotes sales in retail:
- Personalized Marketing Strategies: Data science helps merchants to study client preferences and behavior trends. By exploiting this information, merchants may tailor marketing campaigns and send targeted specials and suggestions to particular consumers. Personalization promotes consumer engagement, builds loyalty, and eventually drives sales.
- Demand Forecasting and Inventory Management: Data science tools, such as predictive analytics, assist retailers in properly estimating demand and optimizing inventory levels. By knowing client preferences, past sales data, and external circumstances, businesses can guarantee they have the correct items in stock at the right time. This decreases stockouts, lowers overstocking, and enhances overall operational efficiency.
- Price Optimization: Data science helps merchants adjust price tactics to promote sales. By evaluating market trends, rival pricing, and consumer data, merchants can establish the most successful price points, discounts, and promotions. This helps increase revenue and attract price-sensitive clients without jeopardizing profitability.
- Customer Segmentation: Data science helps merchants to segment consumers based on multiple characteristics such as demographics, behavior, and purchase history. By splitting consumers into various groups, merchants may establish targeted marketing campaigns and customize product offers to certain categories. This tailored strategy promotes client pleasure, and engagement, and eventually generates sales.
- Recommender Systems: Data science underpins recommender systems, which recommend items or services based on client preferences and historical behavior. These systems may be deployed on e-commerce websites, mobile applications, or in-store displays, increasing the customer’s buying experience and generating more sales via tailored suggestions.
Common applications of data science in retail
Data science has become more crucial in the retail sector, allowing organizations to harness data-driven insights to optimize many parts of their operations. Here are some frequent uses of data science in retail:
- Customer Analytics: Data science helps merchants understand their consumers better. By analyzing consumer data, such as purchase history, browsing activity, demographics, and social media interactions, merchants may determine client preferences, trends, and segments. This intelligence allows individualized marketing campaigns, targeted discounts, and tailored product suggestions to boost consumer happiness and drive sales.
- Price Optimization: Data science helps merchants improve price strategies with sophisticated analytics. By examining market dynamics, rival pricing, historical sales data, and consumer behavior, businesses may establish appropriate price points, discounts, and promotions. Pricing optimization helps optimize revenue, increase competitiveness, and attract price-sensitive consumers without losing profitability.
- Inventory Management: Data science tools, such as demand forecasting and inventory optimization, aid merchants in managing their inventory successfully. By examining past sales data, market trends, seasonal patterns, and external variables, merchants may precisely estimate demand and optimize inventory levels. This guarantees that the correct items are available at the right time, minimizing stockouts, decreasing extra inventory, and lowering operating expenses.
- Market Basket Analysis: Data science allows merchants to undertake market basket analysis, which discovers links and linkages between items usually bought together. By analyzing transactional data, businesses may find trends, propose comparable goods, and improve product placement inside shops or on e-commerce platforms. Market basket research helps raise average transaction values and optimize the consumer purchasing experience.
- Fraud Detection: Data science plays a significant role in preventing and identifying fraud in retail. By examining transactional data, consumer behavior, and network patterns, businesses may spot abnormalities and flag questionable behaviors, such as fraudulent purchases or account takeovers. Fraud detection algorithms assist in limiting financial losses, securing consumer data, and maintaining trust and security.
- Supply Chain Optimization: Data science helps merchants improve their supply chain operations. By examining data linked to suppliers, transportation, inventory levels, and demand trends, retailers may find inefficiencies, cut costs, improve logistics, and boost overall supply chain efficiency. Supply chain optimization assures timely product delivery, reduces interruptions, and enhances customer satisfaction.
Become an Expert on AI Vs Machine Learning Vs Data
Challenges and limitations of using data science in retail
While data science brings various advantages to the retail business, there are also problems and constraints that should be recognized. Here are some of the primary obstacles and constraints of employing data science in retail:
- Data Quality and Accessibility: One key difficulty is the quality and accessibility of data. Retailers may confront challenges with inadequate, inconsistent, or erroneous data, reducing the dependability of analysis and insights gained. Additionally, integrating data from diverse sources and legacy systems may be hard and time-consuming, necessitating data cleansing and standardization activities.
- Privacy and Ethical problems: As merchants gather and analyze vast volumes of consumer data, privacy and ethical problems emerge. Retailers must maintain compliance with data privacy requirements, preserve sensitive consumer information, and get necessary permission for data use. Balancing personalization and privacy is vital to creating and keeping consumer confidence.
- Skill Gap and Talent Acquisition: Data science demands specific skills and knowledge. However, there is a scarcity of trained data scientists in the labor market. Retailers may struggle to locate and hire employees with essential expertise in data analytics, machine learning, and statistical modeling. Upskilling current staff and establishing data-driven cultures become crucial in solving this problem.
- Implementation and Integration: Implementing data science solutions in retail contexts may be complicated. Integrating analytics tools and models into current systems and processes takes careful design and collaboration. Retailers must ensure that data science activities match with their broader business goals and have proper backing from IT departments.
- Changing Consumer Behavior: Consumer behavior is always shifting, and prior data may not always properly anticipate future patterns. Market dynamics and client preferences may change swiftly, making it tough to depend only on past data for decision-making. Retailers need to regularly change their data science strategy to remain ahead of altering customer preferences and industry trends.
- Cost and Return on Investment (ROI): Implementing data science efforts and maintaining the requisite infrastructure may be expensive for retailers, particularly smaller enterprises. It may take a while to achieve the full ROI of data science expenditures. Retailers need to thoroughly examine the costs, advantages, and anticipated returns before starting on data science programs.
- Human Judgment and Interpretation: While data science gives useful insights, human judgment and interpretation are still essential. Data science models and algorithms make suggestions, but they may not capture all essential elements. Retailers need to combine the use of data-driven insights with their sector experience and intuition.
Despite these hurdles and restrictions, data science is a valuable tool for retailers to drive company development, improve consumer experiences, and stay competitive. Addressing these difficulties via rigorous data governance, workforce development, and strategic planning may help retailers leverage the advantages of data science in the retail business.
Implementing data science in retail: Key considerations
Implementing data science in the retail business takes considerable preparation and thought. Here are some crucial concerns for merchants wishing to begin data science initiatives:
- Define Clear Business Objectives: Start by determining the exact business goals you wish to accomplish with data science. Whether it’s boosting client retention, optimizing pricing tactics, or better inventory management, having defined objectives can drive your data science efforts and guarantee alignment with your entire company plan.
- Data Strategy and Infrastructure: Develop a strong data strategy that encompasses data collecting, storage, integration, and security. Determine what data is important to your goals and implement procedures to assure data quality and accessibility. Assess if your present infrastructure can support data science projects or whether improvements and extra resources are necessary.
- Talent Acquisition and Skill Development: Building a professional data science team is vital. Evaluate your present workforce’s competencies and evaluate whether extra talent is required. Hire data scientists with experience in retail analytics, machine learning, and statistical modeling. Support continuing skill development via training programs or collaborations with academic institutions to keep your workforce up to speed with the newest technologies and practices.
- Data Governance and Privacy: Develop clear data governance rules and practices to guarantee compliance with data privacy standards and secure consumer information. Implement suitable data anonymization mechanisms and secure required consents for data collection and use. Establish rules for data retention and destruction to reduce privacy issues.
- Technology and Tools: Evaluate and pick relevant data science tools and technologies that correspond with your company objectives and data architecture. Consider considerations such as scalability, simplicity of integration, user-friendliness, and cost-effectiveness. Leverage cloud-based solutions, open-source platforms, and commercial applications to make data analysis and modeling more efficient.
- Cooperation and Communication: Data science efforts should require cooperation amongst multiple departments, including IT, marketing, operations, and finance. Foster a culture of cooperation and good communication to guarantee that data-derived insights are properly turned into practical plans. Encourage cross-functional teams and frequent contacts to exchange information and foster data-driven decision-making.
- Agile and Iterative strategy: Implement data science efforts using an agile and iterative process. Start with smaller pilot projects or proof of ideas to examine the viability and effect of data science solutions. Learn from the outcomes, receive feedback, and iterate on your models and techniques to constantly improve and iterate over time.
- Monitor effectiveness and ROI: Establish key performance indicators (KPIs) to monitor the effectiveness and impact of your data science activities. Monitor and analyze the performance of your models, monitor key indicators, and measure their influence on business results. Regularly assess and analyze the ROI to verify that your data science activities are producing meaningful value for your firm.
By examining these essential elements, retailers may create a solid basis for the effective adoption of data science and harness its possibilities to promote development and competitive advantage in the retail business
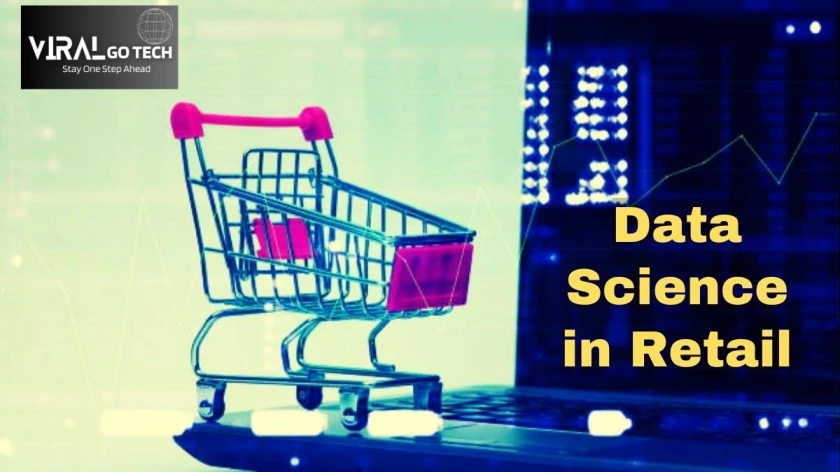
Case studies: Successful examples of data science in retail
Here are a few successful examples of data science applications in the retail industry:
- Amazon’s Personalized Recommendations:
One of the most well-known examples is Amazon’s implementation of personalized product recommendations. By analyzing customer browsing behavior, purchase history, and demographic data, Amazon’s recommendation engine suggests relevant products to individual customers. This personalization has been a key driver of Amazon‘s success, leading to increased sales and customer satisfaction. - Walmart’s Demand Forecasting:
Walmart, one of the largest retail chains globally, uses data science for demand forecasting and inventory management. Through analyzing historical sales data, weather patterns, social media trends, and other factors, Walmart accurately predicts demand for various products. This helps them optimize their inventory levels, reduce stockouts, and improve supply chain efficiency. - Starbucks’ Store Location Optimization:
Starbucks uses data science to identify optimal store locations. By analyzing demographic data, foot traffic patterns, and proximity to competitors, Starbucks can predict the potential success of new store locations. This data-driven approach allows them to strategically expand and maximize their store network’s profitability. - Tesco’s Customer Segmentation:
Tesco, a leading UK supermarket chain, utilized data science to segment its customer base. By analyzing purchasing habits, Tesco identified distinct customer groups with different preferences. This data-driven customer segmentation allowed for targeted marketing campaigns, personalized offers, and improved customer loyalty. - Stitch Fix’s Personalized Styling:
Stitch Fix, an online personal styling service, leverages data science to provide personalized fashion recommendations. By collecting data on customer preferences, body measurements, and feedback on previous items, Stitch Fix’s algorithms generate personalized clothing selections for each customer. This combination of data science and human stylist expertise has led to high customer satisfaction and repeat purchases.
These examples demonstrate how data science drives success in the retail industry by enhancing personalization, optimizing operations, improving decision-making, and ultimately driving sales and customer loyalty. Retailers can learn from these case studies and adapt data science techniques to their specific business models to gain a competitive advantage.
Tools and technologies for data science in retail
There are different tools and technologies available to help data science activities in the retail business. Here are some regularly used ones:
- Python: Python is a popular programming language for data science owing to its vast libraries and packages particularly built for data analysis and machine learning. Libraries like as NumPy, Pandas, and scikit-learn provide significant features for data manipulation, analysis, and modeling.
- R: R is another programming language often used in data science. It contains a full set of tools for statistical analysis, data visualization, and machine learning. R’s versatility and broad libraries make it suited for a wide variety of data science activities in retail.
- SQL: Structured Query Language (SQL) is vital for data capture, data processing, and data integration. SQL helps retail firms to extract, convert, and load data from numerous databases and sources, allowing fast analysis and modeling.
- Apache Hadoop: Hadoop is a system that permits distributed processing and storage of massive datasets across a cluster of computers. It delivers scalability and fault tolerance, making it perfect for processing and analyzing massive data in retail. Hadoop’s ecosystem comprises components including HDFS, MapReduce, and Hive, which are frequently used in data science applications.
- Apache Spark: Spark is a fast and distributed processing engine that can handle large-scale data processing and analytics. It includes tools and APIs for data manipulation, machine learning, and graph processing. Spark is frequently utilized for real-time analytics, recommendation systems, and large-scale data processing in retail.
- Tableau: Tableau is a strong data visualization and business intelligence tool that allows retail organizations to generate interactive dashboards and reports. It lets users analyze data, spot patterns, and convey ideas efficiently. Tableau can connect to many data sources, making it simpler to analyze and display data from multiple systems.
- TensorFlow and Keras: TensorFlow is an open-source deep learning framework created by Google. It offers tools and resources for creating and training neural networks. Keras is a high-level deep learning API that works on top of TensorFlow, simplifying the process of developing and training deep learning models. These technologies are extensively used in retail for tasks like as picture recognition, natural language processing, and anomaly detection.
- DataRobot: DataRobot is an automated machine learning platform that lets firms design and deploy machine learning models fast. It automates many phases of the modeling process, including data pretreatment, feature selection, and model validation. DataRobot streamlines the development and deployment of machine learning models in retail.
The choice of tools relies on unique needs, data complexity, and the competence of the data science team. Retailers should carefully examine and pick the solutions that best correspond with their data science objectives and infrastructure.
Future of data science in retail
The future of data science in retail is bright. As the retail business continues to grow, data science will become more vital. Here are some of the ways that data science is predicted to be applied in retail in the future:
- Real-time personalization: Retailers will employ data science to customize the shopping experience for consumers in real time. This might entail suggesting goods that clients are likely to be interested in, or giving discounts and promotions based on their present location or purchasing history.
- Augmented reality and virtual reality: Data science will be employed to fuel augmented reality (AR) and virtual reality (VR) experiences in retail. This may entail letting clients try on clothing or cosmetics online, or see how furniture would appear in their house before they purchase it.
- Blockchain: Data science will be leveraged to build blockchain-based solutions in retail. This might entail tracing the source of items or managing supply networks in a more effective manner.
- Artificial intelligence: Artificial intelligence (AI) will play a crucial role in the future of data science in retail. AI will be used to automate operations, such as customer support and fraud detection, and to create predictions about client behavior.
- Edge computing: Edge computing will enable merchants to process data closer to the point of sale. This will increase the speed and accuracy of data processing, and it will also assist in minimizing latency.
Overall, the future of data science in retail is highly bright. As the technology continues to grow, retailers will discover even more imaginative ways to employ data science to enhance the shopping experience for consumers and to gain a competitive advantage.
Conclusion:
In conclusion, utilizing the potential of data science in retail is no longer simply a competitive advantage, but a necessity in today’s digital world. By leveraging modern analytics methods and technology, retailers may avail themselves of the amount of data at their disposal to streamline operations, tailor marketing campaigns, and ultimately increase their bottom line. From anticipating client behavior to offering customized items, the potential of data science in the retail industry is vast. Embracing this disruptive technology is crucial for shops that aim to remain ahead of the competition and deliver a great customer experience. So, let us harness the full potential of data science and go on a path of success in the world of retail.